The Economics of Artificial Intelligence: Focusing on the Metrics
Images
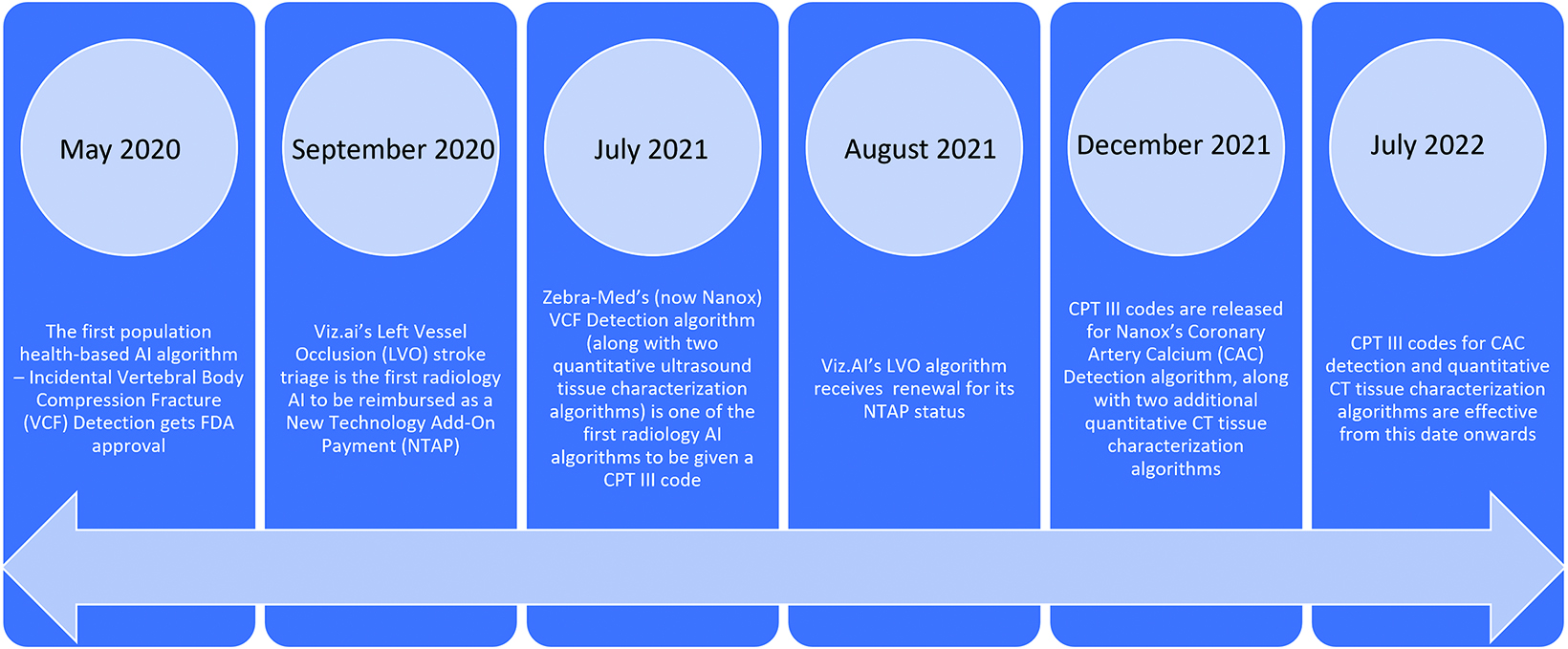
In the 20th century, medical imaging and artificial intelligence (AI) developed in parallel. They converged at the turn of the century with US Food and Drug Administration (FDA) approval of a computer-aided detection system for breast microcalcifications.1 After a lull in development, recent breakthroughs in machine learning and deep learning technologies have given way to a renaissance of AI in radiology. Within the past decade, AI has been widely adopted in radiology practices. This can be attributed in part to the ability of newer AI tools to learn, allowing them to be integrated into the radiology workflow. Healthcare AI tools have different parameters for judgment as medical devices: development is faster, modifications are greater, and access to real world data is wider. These differences, along with the anticipation of a substantial increase in AI-related submissions for FDA premarket approval, have encouraged the FDA to update its existing approval framework to accommodate these new technologies.2
Reimbursement of AI Algorithms: How Are They Valued?
As AI advances, radiology’s growing adoption of and reliance on AI tools raises a question: How do practices pay for them? In the development phase, vendors collaborate with radiologists and subsequently typically provide their tools on a subscription basis. In recent years, the Centers for Medicare and Medicaid (CMS) has acknowledged the value of these tools by providing several new reimbursement opportunities. In September 2020, for example, Viz.AI’s Large Vessel Occlusion AI algorithm made headlines as the first in radiology to be reimbursed as a New Technology Add-On Payment (NTAP), and its status was renewed in August 2021.3
The first population health-based AI algorithm, Incidental Vertebral Body Compression Fracture (VCF) detection on chest CT scans (by Zebra Medical Vision, now Nanox), received FDA approval in May 2020. In July 2021, the VCF algorithm had its landmark moment: it was one of three radiology algorithms to have ever been given a Current Procedural Terminology (CPT) code (the others being related to quantitative ultrasound tissue characterization). Additional CPT codes for another population health-based algorithm centered on coronary artery calcium detection, and two codes for quantitative CT characterization were released in December 2021 and will become effective in July 2022 (Figure).4
All these algorithms have been given a CPT Category III code, which has no relative value unit valuation and is not reimbursed by CMS at the national level. CPT Category III codes are bundled with Category I CPT codes and are used to identify clinically effective new technologies and services.
As described by the American Medical Association, technologies and services with CPT Category III codes could transition to reimbursable CPT Category I codes if they satisfy the following criteria within five years:
- They received FDA clearance or approval, when such is required for performance of the procedure or service;
- They are performed by many physicians or other qualified healthcare professionals across the United States;
- They are performed with frequency consistent with the intended clinical use (ie, a service for a common condition should have high volume);
- They are consistent with current medical practice; and,
- Their clinical efficacy is documented in literature that meets the requirements set forth in the CPT code-change application5
Several algorithms demonstrate these criteria and upon widespread adoption will have a good chance of becoming eligible for a reimbursable CPT Category I code. Direct reimbursement will encourage more healthcare systems and radiologists to adopt them. But the question arises: Should direct reimbursement be the sole metric in considering when to choose an AI algorithm?
Considering AI’s value to radiologists from a different perspective is equally important. Radiology AI algorithms should be reimbursed not only through fee-for-service payments, but also through value-based models. In quality circles, the value equation is used to define the value of a tool. An increase in value can be achieved by improving outcomes and/or reducing costs, as illustrated by this equation:
Value = Quality of Outcomes/Cost6
Radiologists and patients are the primary stakeholders with respect to radiology AI algorithm use; anything that can be done to increase its value to either party is desirable.
The Value of AI Algorithms to Radiologists
The numerous varieties of AI algorithms available, in use, and in development will assist radiologists in several aspects of their job.
For example, diagnostic AI algorithms (eg, those that facilitate detection of intracranial hemorrhages, pulmonary emboli, and fractures) are effective. They alert radiologists to potential positive findings on a worklist that would have otherwise been read chronologically. This can lead to faster diagnoses and more rapid notification of the appropriate health team, thereby improving patient management. Early diagnosis through efficient and accurate scan interpretations also results in lower patient morbidity, reducing costs to the healthcare facility. A randomized controlled trial found that emergency department patients in the immediate reporting arm, where scan interpretations were delivered in shorter time intervals, had fewer ED recalls and lower rates of short-term inpatient bed days.7
AI-based algorithms can also reduce errors and their inherent risk of legal consequences. Radiologists are under growing pressure to interpret larger volumes of scans at faster rates. As of 2010, the number of computed tomography (CT) examinations in a single institution increased 1300% over an 11-year period, leading to a sevenfold increase in a single radiologist’s workload. It was calculated that the average radiologist in the institution was interpreting one image approximately every four seconds in an eight-hour workday, leading to an increased risk for errors and burnout.8
Indeed, a 2015 pilot study found that radiologists who read scans at twice their normal speed had an average interpretation error rate of 26.6%, as compared to an error rate of 10% at their normal speed.9 A retrospective analysis of diagnostic errors recorded over a 92-month period in a hospital in Auckland, New Zealand, found that 80% of these errors were perceptual; ie, they resulted from a failure in detection.10
All this is noteworthy in light of the fact that the most common cause of medical malpractice suits against radiologists in the United States are diagnostic errors, which account for 14.83 claims filed per 1000 person-years.11
It is clear that AI algorithms that focus on improving logistics and streamlining workflow, including study ordering and acquisition, radiologist scheduling, report generation, patient notification, and peer review, demonstrate their value by improving diagnostic accuracy and report turnaround times.
They can even improve radiologist well-being. A 2016 report by the American College of Radiology Commission of Human Resources recognized that radiologist burnout was the seventh-highest among all physicians and identified heavy workloads and severe time constraints as contributing factors. The ACR report recommended development of efficient workflows as one strategy to combat this burnout, a strategy to which AI-based algorithms can certainly contribute.12
The Value of AI Algorithms to Patients
AI-based radiology algorithms can also benefit patients through their ability to enhance report quality and reduce turnaround times; ie, patients stand a greater chance of getting a faster diagnosis and treatment plan. The burgeoning field of opportunistic screening has the potential to add value from a population health perspective. Breast cancer screening programs have shown that regular mammography examinations reduce rates of morbidity. One study found that women who participated in screening had a 41% reduced risk of breast cancer-related death within 10 years, and a 25% reduction in their rate of advanced breast cancer.13
AI-based population health tools provide incidental information on studies and proactively manage patient health. These algorithms can identify high-risk patients and aid in developing a systematic workflow directing them to appropriate preventive care. This will reduce the need for more costly ED and inpatient services. There is also great potential for other types of opportunistic imaging to advance preventive health care while simultaneously reducing downstream healthcare costs.
What Metrics Should Be Influencing Our Choices?
Over time, AI-based algorithms for radiology will continue to improve, and their value will continue to rise. From an economic standpoint, CMS recognition of these algorithms for direct reimbursement is important to consider when deciding whether to adopt them. However, financial value should not outbalance other criteria, such as the impact of a given tool on the quality of radiologist performance and patient care, which are equally important. The burden of expense should not hinder utilization and further development of additional tools that can aid in patient management.
References
- Do YA, Jang M, Yun BL et al. Diagnostic performance of artificial intelligence-based computer-aided diagnosis for breast microcalcification on mammography. Diagnostics (Basel). 2021;11(8):1409. doi: 10.3390/diagnostics11081409.
- Diamond M. Presentation: Proposed regulatory framework for modifications to artificial intelligence/machine learning. 2020. https://www.fda.gov/media/135713/download. Accessed March 7, 2022.
- Chen MM, Golding LP, Nicola GN. Who will pay for AI? Radiol Artif Intell. 2021;3(3):210030. doi: 10.1148/ryai.2021210030.
- “cpt-category3-codes-long-descriptors.pdf.” 2022. https://www.ama-assn.org/system/files/cpt-category3-codes-long-descriptors.pdf. Accessed March 1, 2022.
- Criteria for CPT® Category I and Category III Codes. American Medical Association. https://www.ama-assn.org/practice-management/cpt/criteria-cpt-category-i-and-category-iii-codes. Accessed February 17, 2022.
- Weeks BW, Weinstein JN. Caveats to consider when calculating healthcare value. Am J Med. 2015;128(8):802-803. doi: 10.1016/j.amjmed.2014.11.012
- Hardy M, Snaith B, Scally A. The impact of immediate reporting on interpretative discrepancies and patient referral pathways within the emergency department: a randomised controlled trial. Br J Radiol. 2013;86(1021):20120112. doi: 10.1259/bjr.20120112
- McDonald RJ, Schwartz KM, Eckel LJ et al. The effects of changes in utilization and technological advancements of cross-sectional imaging on radiologist workload. Acad Radiol. 2015;22(9):1191-1198. doi: 10.1016/j.acra.2015.05.00.
- Sokolovskaya E, Shinde T, Ruchman BR, et al. The effect of faster reporting speed for imaging studies on the number of misses and interpretation errors: a pilot study. J Am Coll Radiol. 2015;12(7):683-688. doi:10.1016/j.jacr.2015.03.040.
- Donald JJ, Bernard SA. Common patterns in 558 diagnostic radiology errors. J Med Imaging Rad Oncol. 2012; 56(2):173-178. doi: 10.1111/j.1754-9485.2012.02348.x
- Whang JS, Baker SR, Patel R, et al. The causes of medical malpractice suits against radiologists in the United States. Radiol. 2013;266(2):548-554. doi: 10.1148/ radiol.12111119.
- Harolds JA, Parikh JR, Bluth EI. Burnout of radiologists: frequency, risk factors, and remedies: a report of the ACR Commission of Human Resources. J Am Coll Radiol. 2016;4(4):411-416. doi: 10.1016/j.jacr2015.11.003
- Duffy SW, Tabar L, Yen AM, et al. Mammography screening reduces rates of advanced and fatal breast cancers: Results in 549,091 women. Cancer. 2020;126(13):2971-2979. doi: 10.100 2/cncr.32859.
References
Citation
M K, RK L,. The Economics of Artificial Intelligence: Focusing on the Metrics. Appl Radiol. 2022;(3):13-15.
April 26, 2022